This essay is part of the series: AI F4: Facts, Fiction, Fears and Fantasies.
The phenomenon of anthropomorphisation has emerged as a critical topic for discussion and action as generative AI becomes more pervasive. Anthropomorphisation, or attributing human characteristics to AI, can improve user interaction and trust in systems. Still, it has potential negative consequences, such as misleading users about the capabilities of the AI and raising ethical and privacy concerns.
Anthropomorphisation has been depicted dramatically in movies, the most famous example of which is Terminator in embodied form with less popular examples including the smart voice assistant in H.E.R. Such depictions vary in different parts of the world, for example, Astroboy in Japan is a friendlier and more culturally-attuned example, while the others mentioned here centre a lot more on North American cultural dispositions. This has had an impact on the development and perception of robotics, with higher acceptance, for example, of social care robotics in nursing homes for the elderly in Japan while being eschewed in the Western world.
Anthropomorphisation in AI goes beyond mere user perception; it influences how these systems are designed, marketed, and integrated into societal structures.
Gaining a better understanding of the dynamics and underlying assumptions will help us steer the future toward alignment with our cultural aspirations and values, localised and adapted to meet differing hopes and fears. Anthropomorphisation in AI goes beyond mere user perception; it influences how these systems are designed, marketed, and integrated into societal structures. The tendency to perceive AI as human-like can lead to unrealistic expectations, ethical dilemmas, and even legal challenges.
A framework for tackling the challenges posed by anthropomorphisation
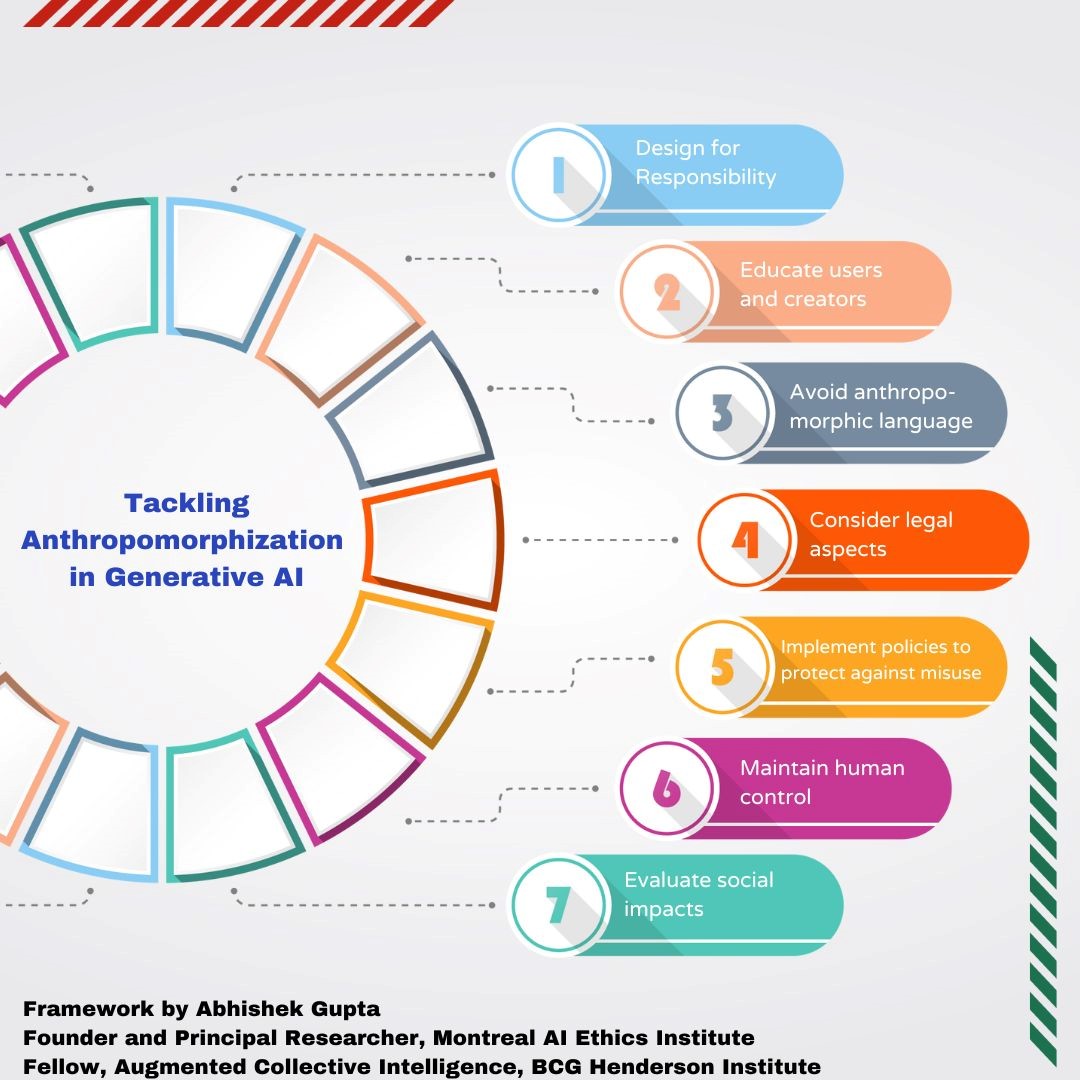
To tackle the social and technical aspects of the challenges posed by anthropomorphisation, this article proposes a framework that consists of 7 pillars:
- Design for responsibility: Identify and document potential harms at the start of the generative AI product development process. This includes considering the potential for anthropomorphisation and its effects.
- Educate users and creators: Both users and creators of AI systems should be educated about the potential consequences of anthropomorphisation.
- Avoid anthropomorphic language: The language used to describe AI and robotic systems should be chosen carefully to avoid creating false expectations and assumptions about the capabilities of these systems.
- Consider legal aspects: The legal aspects of anthropomorphisation should be considered, particularly in the context of existing laws and regulations.
- Implement policies to protect against misuse: Policies should be implemented to protect against the misuse and abuse of generative AI, including measures to prevent deceptive or unfair practices.
- Maintain human control: Generative AI should be viewed as a tool to support human creators rather than an agent capable of harbouring its own intent or authorship. This includes maintaining meaningful human control in the context of generative AI.
- Evaluate social impact: The social impact of generative AI systems should be evaluated, including the potential effects of anthropomorphisation.
Each of these seven pillars can be used to look at the real-world applications of generative AI.
Adobe's generative AI models, which learn by scrubbing the internet, have raised concerns among artists and illustrators. These models can use, break apart, rearrange, and regenerate artists' work without permission or attribution, violating copyright laws and personal styles. On the other hand, Google has implemented an ethics review process in developing their AI products, including generative AI. They proactively integrate ethical considerations early in the design and development process, focusing on guidance around generative AI projects. This demonstrates the pillar of “Designing for Responsibility” whereby proactive identification and documentation of the workings of the systems can lead to drastically different perceptions and outcomes.
Adobe's generative AI models, which learn by scrubbing the internet, have raised concerns among artists and illustrators.
As AI systems pervade more and more aspects of our lives, for example, in the healthcare sector, patients often need to share sensitive information with AI systems without being fully aware of the consequences of such actions. This lack of education can lead to loss of privacy and potential errors or injuries due to miscalculations of the system. On the other hand, for example, OpenAI has been working on making its AI model, ChatGPT, safer and less biased. They have developed tools to help artists protect their work from AI art generators, educating creators about the potential misuse of their work.
As much as we’d like (or perhaps not) for AI systems to behave like a human conversation partner, sometimes a mismatch between what the system actually is and what we expect it to be can cause issues. For example, a hallucinating chatbot which ends up misleading users about its capabilities. On the other hand, in the retail industry, generative AI is used for personalised recommendations and trend analysis. The language used to describe these AI systems is carefully chosen to avoid creating false expectations about their capabilities. In the latter case, the organisation can achieve better business outcomes from their investments into building these systems as opposed to creating issues of distrust due to miscalibration which can happen when we avoid the use of anthropomorphic language.
In the financial sector, banks like BBVA have taken a conservative approach to AI, considering the legal aspects of handling large volumes of sensitive customer data.
Given the push around the world in bringing to bear legal and regulatory requirements in how AI systems are developed and deployed, considering legal aspects is an essential pillar in the framework. For example, in the financial sector, banks like BBVA have taken a conservative approach to AI, considering the legal aspects of handling large volumes of sensitive customer data. But, as we saw earlier, Generative AI models used by Adobe have raised legal concerns about copyright infringement and attribution.
Since modern AI systems, especially when we start to anthropomorphise them, can tap into issues of algorithmic aversion and automation bias tendencies, it is important that we implement policies to protect against misuse. Google has implemented best practices for developing generative AI, including using responsible datasets, classifiers, and filters. Failure to do so can lead to significant problems, especially in high-stakes scenarios. In the healthcare sector, generative AI systems have been found to produce incorrect responses frequently, requiring human intervention to ensure beneficial suggestions for patients. This indicates ineffective policies to protect against misuse.
Building on the above push to prevent misuse, maintaining human control is another important consideration when seeking to combat the problem of anthropomorphisation since it can help dispel fascination with these systems without fully understanding how they function. In the healthcare sector, generative AI systems are seen as tools to augment the efforts of human clinicians rather than replace them. This perspective maintains meaningful human control in the context of generative AI. Additionally, in the financial sector, generative AI has been used to automate complex processes. However, the technology is still in its early days, and many banks have cautioned that it may be too risky to implement it in areas that touch consumers, indicating a lack of human control.
Google has implemented best practices for developing generative AI, including using responsible datasets, classifiers, and filters. Failure to do so can lead to significant problems, especially in high-stakes scenarios.
Finally, we’d be remiss if we didn’t evaluate the social impact of the deployment of such systems, especially given that AI systems are sociotechnical in nature, and anthropomorphisation makes them more so. In the retail industry, Generative AI systems have been used to offer visual search and virtual try-on experiences, enhancing customer engagement and transforming the shopping experience. This offers personalisation and helps small businesses retain customers while minimising costs. On the flip side, solely focusing on business outcomes can have an overall negative impact. Generative AI models used by Adobe have raised concerns about bias and lack of diversity, indicating a failure to evaluate and address the social impact of these systems.
Navigating the AI frontier
So, what can professionals working in the field do to proactively manage these concerns?
- Embrace responsible design: AI practitioners must start by incorporating ethical considerations from the outset of any project. This involves identifying potential harms and consciously designing to minimise the risk of anthropomorphisation.
- Educational outreach: Both creators and users of AI systems should be educated about the nuances of anthropomorphisation. This includes understanding the limitations of AI and the potential ethical implications of its use.
- Language and communication: Carefully choose the language used to describe AI systems. Avoid anthropomorphic language that could create unrealistic expectations about the capabilities of these systems.
- Legal and regulatory compliance: Stay abreast of evolving legal landscapes concerning AI. Understand and comply with regulations to avoid legal pitfalls related to anthropomorphisation.
- Policy development for misuse prevention: Develop and implement policies to safeguard against the misuse of AI. This includes setting up frameworks to prevent deceptive practices and ensuring the ethical use of AI.
- Prioritise human oversight: Maintain a perspective of AI as a tool to augment human abilities, not replace them. This involves ensuring meaningful human control in the deployment of AI systems.
- Assess social impact: Regularly evaluate the social impact of AI systems. This includes understanding how these systems affect different demographics and taking steps to address any negative consequences.
Utilising a holistic framework instead of going at these challenges in an ad-hoc fashion will ensure that we harness the potential that AI systems have to offer while mitigating the downsides that they inevitably pose.
Abhishek Gupta is the Founder and Principal Researcher, Montreal AI Ethics Institute.
The views expressed above belong to the author(s). ORF research and analyses now available on Telegram! Click here to access our curated content — blogs, longforms and interviews.